The call center industry stands at a pivotal crossroads. For decades, these vital customer service hubs have served as the primary interface between businesses and their customers, handling everything from routine inquiries to complex problem-solving. Today, however, artificial intelligence is fundamentally transforming this landscape at an unprecedented pace, creating both extraordinary opportunities and significant challenges for organizations worldwide.
The global call center industry, valued at approximately $339.4 billion in 2024, represents a massive ecosystem spanning diverse geographies from North America to Asia. In the United States alone, call centers employ over 2.8 million people, while countries like India and the Philippines have built substantial economies around business process outsourcing, with call centers at their core. This industry has traditionally been characterized by high turnover rates, significant training costs, and the constant challenge of balancing quality customer experiences with operational efficiency.
Artificial intelligence has emerged as a transformative force in this space, promising to address many of these longstanding challenges. From intelligent virtual assistants that can handle routine inquiries to sophisticated analytics that provide real-time guidance to human agents, AI technologies are reshaping every aspect of call center operations. The integration of machine learning, natural language processing, and predictive analytics is enabling organizations to deliver more personalized, efficient, and effective customer service experiences.
The urgency of understanding and adapting to these changes cannot be overstated. According to recent research, 85% of customer interactions are expected to be handled without human agents by 2026. Organizations that fail to embrace AI-driven transformation risk falling behind competitors who can deliver superior customer experiences at lower costs. Meanwhile, those that implement AI without careful consideration of the human element may alienate both customers and employees, ultimately undermining the very relationships they seek to strengthen.
This article explores the multifaceted impact of AI on call centers, examining current applications, emerging technologies, and strategic considerations for organizations navigating this complex landscape. By understanding both the technological possibilities and the human dimensions of this transformation, business leaders can develop thoughtful approaches that leverage AI to enhance rather than replace the human connections that remain essential to meaningful customer service.
Current State of AI in Call Centers
The Evolution of Call Center Technology
The call center industry has undergone a remarkable evolution over the past decade, transitioning from traditional voice-centric operations to sophisticated multi-channel engagement hubs powered by artificial intelligence. This transformation has been driven by changing customer expectations, competitive pressures, and technological advancements that have made AI more accessible, affordable, and effective for organizations of all sizes.
Historically, call centers relied heavily on human agents supported by basic technologies like interactive voice response (IVR) systems and automatic call distributors (ACDs). These systems provided rudimentary automation but often created frustrating customer experiences characterized by lengthy menus, limited self-service options, and poor routing accuracy. Today’s AI-powered call centers represent a quantum leap forward, leveraging sophisticated algorithms to understand natural language, predict customer needs, automate routine tasks, and augment human capabilities.
Key AI Technologies Transforming Call Centers
Several core AI technologies are currently reshaping the call center landscape:
Natural Language Processing (NLP) and Understanding (NLU)
NLP and NLU technologies enable systems to comprehend human language in both written and spoken form. In call centers, these technologies power intelligent virtual assistants and chatbots that can understand customer queries expressed in natural language, interpret intent, and respond appropriately. Advanced NLP systems can detect nuances, handle multiple intents within a single query, and maintain context throughout conversations. This represents a significant improvement over rule-based systems that relied on specific keywords or phrases.
In the United States and Canada, major financial institutions have deployed NLP-powered virtual assistants that can handle over 70% of routine customer inquiries without human intervention. These systems continuously learn from interactions, becoming more accurate and capable over time. Similarly, telecommunications providers in Mexico and Colombia have implemented NLP solutions that support multiple dialects and regional language variations, enabling more inclusive and effective customer service.
Speech Recognition and Voice Analytics
Speech recognition technology has reached unprecedented levels of accuracy, enabling real-time transcription of customer calls with error rates below 5% in optimal conditions. This capability supports numerous applications, from documenting customer interactions to triggering automated workflows based on specific phrases or requests.
Voice analytics goes beyond basic transcription to extract meaningful insights from spoken interactions. These systems can identify customer sentiment, detect escalation triggers, recognize compliance issues, and even identify potential sales opportunities. In India’s expansive call center industry, voice analytics has become a standard tool for quality assurance, automatically evaluating thousands of calls daily against predefined criteria and providing targeted coaching recommendations for agents.
Predictive Analytics and Machine Learning
Predictive analytics leverages historical data to forecast future outcomes, enabling call centers to anticipate customer needs, optimize staffing, and proactively address potential issues. Machine learning algorithms continuously refine these predictions based on new data, creating increasingly accurate models over time.
In practice, these technologies enable capabilities like:
- Predicting which customers are likely to call and why, allowing for proactive outreach
- Identifying which customers are at risk of churn based on interaction patterns
- Forecasting call volumes with greater accuracy to optimize staffing and reduce wait times
- Recommending next-best actions for agents based on similar historical scenarios
The Philippines, as a global leader in business process outsourcing, has seen widespread adoption of predictive analytics platforms that help call centers manage workforce requirements across different time zones and client portfolios, significantly improving operational efficiency.
Robotic Process Automation (RPA)
RPA technology automates repetitive, rule-based tasks that previously required human intervention. In call centers, RPA bots can update customer records, process transactions, generate reports, and integrate information across multiple systems without manual effort. This technology is particularly valuable for back-office operations that support customer-facing interactions.
A major telecommunications provider in Canada reported a 40% reduction in post-call processing time after implementing RPA to automate data entry and verification tasks. Similarly, insurance companies in the United States have deployed RPA to streamline claims processing, allowing agents to focus on customer communication rather than administrative tasks.
Regional Adoption Patterns
AI adoption in call centers varies significantly across regions, influenced by factors including technological infrastructure, labor costs, regulatory environments, and competitive dynamics:
United States and Canada
North American markets have been early adopters of advanced AI technologies, driven by high labor costs and customer expectations for seamless digital experiences. Large enterprises in financial services, telecommunications, and retail have made significant investments in comprehensive AI platforms that integrate multiple technologies to create end-to-end automation capabilities.
A distinctive trend in these markets is the focus on omnichannel integration, with AI systems designed to provide consistent experiences across voice, chat, email, and social media channels. Companies are increasingly implementing “digital-first, but not digital-only” strategies that leverage AI for routine interactions while preserving human connections for complex or emotionally charged situations.
Mexico and Colombia
In Latin America, Mexico and Colombia have emerged as important call center hubs serving both domestic markets and U.S.-based customers. AI adoption in these regions has accelerated in recent years, with particular emphasis on bilingual capabilities that can seamlessly transition between English and Spanish.
Many organizations in these markets have adopted a pragmatic approach to AI implementation, focusing on specific high-value use cases rather than comprehensive transformation. Virtual assistants for first-level customer support, automated quality monitoring, and agent assistance tools have gained significant traction, while more advanced applications like predictive behavioral routing are still in earlier stages of adoption.
India
India’s massive call center industry, which employs millions of workers serving global clients, has embraced AI as both an opportunity and a necessity in the face of evolving client expectations. The country’s strong technical talent pool has enabled sophisticated AI implementations, particularly in large business process outsourcing (BPO) organizations.
Indian call centers have been particularly innovative in developing AI solutions that augment rather than replace human agents. These include real-time guidance systems that provide agents with recommended responses, sentiment analysis tools that flag emotional customers for specialized handling, and automated coaching platforms that deliver personalized training based on individual performance patterns.
Philippines
The Philippines, another global BPO powerhouse, has seen strategic AI adoption focused on enhancing the country’s competitive position in higher-value services. With rising labor costs and competition from other offshore locations, Philippine call centers have leveraged AI to move up the value chain from basic customer support to more complex services.
Voice analytics and quality assurance automation have seen particularly strong adoption, helping organizations maintain consistent service levels across large agent populations. Additionally, AI-powered training and simulation tools have become increasingly common, enabling more efficient onboarding and continuous skill development for agents.
Current Implementation Challenges
Despite significant progress, organizations across all regions continue to face common challenges in AI implementation:
Integration with Legacy Systems
Many call centers operate with complex technology ecosystems built over decades, including customer relationship management (CRM) systems, workforce management platforms, telephony infrastructure, and various specialized applications. Integrating AI capabilities with these legacy systems often requires significant custom development and middleware solutions.
Data Quality and Availability
AI systems require large volumes of high-quality data for training and operation. Organizations frequently struggle with fragmented customer information, inconsistent data formats, and privacy restrictions that limit data accessibility. These challenges are particularly acute in highly regulated industries like healthcare and financial services.
Measurement and ROI Validation
Quantifying the business impact of AI investments remains challenging for many organizations. While metrics like containment rate (percentage of interactions handled without human intervention) and average handling time are relatively straightforward, measuring improvements in customer satisfaction, loyalty, and lifetime value requires more sophisticated analytics capabilities.
Change Management and Workforce Transition
Perhaps the most significant challenge lies in managing the human dimensions of AI adoption. Organizations must help agents adapt to new workflows, develop skills for collaborating effectively with AI systems, and navigate concerns about job security. This requires thoughtful change management strategies, transparent communication, and investment in reskilling programs.
As we move forward, the most successful organizations will be those that address these challenges holistically, recognizing that technological implementation is just one aspect of a broader transformation journey that encompasses people, processes, and organizational culture.
Challenges and Limitations of AI in Call Centers
Technical Challenges
Despite the significant advancements in AI technology for call centers, several technical challenges continue to limit its effectiveness and widespread adoption. Understanding these limitations is crucial for organizations seeking to implement AI solutions successfully.
Inaccurate Responses and AI Hallucinations
One of the most significant technical challenges facing AI systems in call centers is the problem of inaccurate responses and “hallucinations” – instances where AI generates plausible-sounding but factually incorrect information. This issue is particularly prevalent in generative AI models that produce original content rather than selecting from predefined responses.
These inaccuracies can stem from several sources:
- Outdated or incomplete training data: AI systems can only be as good as the data they’re trained on. When information changes or the system encounters scenarios not represented in its training data, it may generate incorrect responses.
- Contextual misunderstanding: AI systems sometimes struggle to maintain context throughout complex conversations, leading to responses that may be technically correct but inappropriate for the specific situation.
- Overconfidence in uncertain situations: Rather than acknowledging uncertainty, some AI systems will generate definitive-sounding but incorrect answers when faced with ambiguous queries.
The consequences of these inaccuracies can be severe, particularly in industries like healthcare, finance, and insurance, where providing incorrect information can have legal implications or negatively impact customer outcomes. Organizations must implement robust verification mechanisms and clear escalation paths to human agents when AI confidence levels fall below acceptable thresholds.
Integration with Legacy Systems
Many call centers operate within complex technological ecosystems built over decades, including customer relationship management (CRM) systems, billing platforms, knowledge bases, and specialized industry applications. Integrating AI capabilities with these legacy systems presents significant challenges:
- Data silos: Critical information may be trapped in disparate systems with limited interoperability, preventing AI from accessing the comprehensive view needed to serve customers effectively.
- Inconsistent data formats: Different systems may store similar information in incompatible formats, complicating the training and operation of AI models that require standardized inputs.
- Real-time access limitations: Legacy systems may not support the real-time data access required for AI to provide immediate, contextually relevant responses during customer interactions.
- Technical debt: Outdated code, poor documentation, and accumulated workarounds in legacy systems can make integration projects unpredictable and costly.
Organizations often find themselves caught between the substantial investment required for complete system modernization and the limitations imposed by attempting to layer AI capabilities onto incompatible infrastructure.
Language and Dialect Limitations
While natural language processing has advanced significantly, AI systems still struggle with the full complexity of human communication, particularly across diverse linguistic contexts:
- Accent and dialect variations: Speech recognition systems may perform inconsistently across different accents and regional dialects, potentially creating disparate experiences for customers from different backgrounds.
- Code-switching and multilingual conversations: Customers who alternate between languages during a single conversation can confuse AI systems not specifically designed to handle such transitions.
- Cultural nuances and idioms: AI may miss culturally specific expressions, metaphors, and contextual cues that human agents would naturally understand.
- Emotional and paralinguistic elements: Tone, pace, volume, and other non-verbal aspects of communication carry significant meaning that AI systems may not fully capture or interpret correctly.
These limitations are particularly relevant for global organizations and those serving diverse customer populations. Companies operating in regions like India, the Philippines, and Latin America, where linguistic diversity is high, must carefully evaluate AI solutions for their ability to handle local language variations effectively.
Operational Challenges
Beyond technical limitations, organizations implementing AI in call centers face significant operational challenges that can impact the success of these initiatives.
Implementation and Change Management
Deploying AI in call centers represents a fundamental transformation of operational processes, requiring careful change management:
- Resistance to change: Agents and supervisors may resist new AI tools due to concerns about job security, performance monitoring, or disruption to familiar workflows.
- Training requirements: Effective collaboration with AI systems requires new skills that existing staff may need time and support to develop.
- Process redesign: Existing call center processes often need substantial revision to incorporate AI capabilities effectively, requiring cross-functional coordination and careful planning.
- Unrealistic expectations: Organizations sometimes implement AI with inflated expectations about immediate cost savings or performance improvements, leading to disappointment and potential abandonment of valuable initiatives.
Successful implementations typically involve extensive stakeholder engagement, realistic timeline setting, and phased approaches that allow for learning and adjustment.
Customer Resistance to Automation
While organizations may be eager to implement AI solutions, customer acceptance remains a significant challenge:
- Preference for human interaction: Recent research indicates that 61% of consumers prefer to complete tasks through human channels, and 74% would rather resolve issues via human agents.
- Declining comfort with AI: Consumer comfort with AI overall has decreased by 11% year-over-year, reflecting growing concerns about privacy, accuracy, and the quality of automated interactions.
- Transparency concerns: Customers may feel uncomfortable when uncertain whether they’re interacting with an AI or human agent, particularly if the AI attempts to present itself as human.
- Frustration with limitations: When AI systems fail to understand requests or provide relevant responses, customer frustration can quickly escalate, potentially damaging brand perception.
Organizations must carefully balance automation opportunities with customer preferences, providing clear options for human escalation and designing experiences that leverage AI appropriately based on the nature and complexity of different customer needs.
Data Privacy and Security Concerns
AI systems in call centers process vast amounts of sensitive customer information, raising significant privacy and security considerations:
- Regulatory compliance: Organizations must navigate complex and evolving regulations like GDPR, CCPA, and industry-specific requirements that govern the collection, storage, and processing of customer data.
- Consent management: Obtaining and maintaining appropriate consent for AI applications, particularly those involving voice recording or biometric data, presents legal and operational challenges.
- Data minimization: AI systems often benefit from access to comprehensive customer information, creating tension with privacy principles that advocate for collecting and retaining only essential data.
- Security vulnerabilities: The integration of AI systems may introduce new security risks, particularly when these systems require access to multiple data sources across the organization.
These concerns are particularly acute in highly regulated industries like healthcare and financial services, where additional requirements around data handling may apply.
Strategic Challenges
At the strategic level, organizations face fundamental questions about how AI should transform their call center operations and customer experience approach.
Balancing Automation and Human Touch
Perhaps the most significant strategic challenge is determining the appropriate balance between automation and human interaction:
- Customer experience trade-offs: While AI can improve efficiency and consistency, it may struggle to deliver the empathy, emotional intelligence, and creative problem-solving that human agents provide in complex or emotionally charged situations.
- Brand alignment: The extent and nature of AI deployment should align with brand positioning and customer expectations. Premium brands may need to emphasize human interaction, while efficiency-focused brands might lean more heavily on automation.
- Value differentiation: As AI capabilities become more standardized across the industry, organizations must consider how their approach to balancing technology and human elements creates distinctive value for customers.
- Ethical considerations: Decisions about automation inevitably raise questions about organizational values and responsibilities toward both customers and employees.
There is no universal answer to these questions – each organization must determine the right balance based on their specific context, customer needs, and strategic objectives.
Measuring ROI and Performance
Quantifying the business impact of AI investments in call centers presents significant challenges:
- Attribution complexity: Improvements in metrics like customer satisfaction or retention typically result from multiple factors, making it difficult to isolate the specific contribution of AI implementations.
- Indirect benefits: Many AI benefits, such as improved agent satisfaction or enhanced brand perception, may not immediately appear in financial metrics but can significantly impact long-term business performance.
- Evolving metrics: Traditional call center metrics like average handling time may become less relevant as AI transforms the nature of customer interactions, requiring new measurement approaches.
- Investment timeframes: The full benefits of AI implementations often emerge over extended periods as systems learn and improve, creating challenges for traditional ROI calculations with fixed evaluation periods.
Organizations need sophisticated measurement frameworks that capture both immediate operational impacts and longer-term strategic benefits to evaluate AI investments accurately.
Workforce Transformation
AI implementation inevitably raises questions about the future composition and capabilities of the call center workforce:
- Skill evolution: As AI handles routine interactions, human agents need enhanced skills in complex problem-solving, emotional intelligence, and technology collaboration.
- Role redesign: New positions emerge at the intersection of technology and customer service, requiring organizations to create career paths that reflect these evolving requirements.
- Recruitment implications: The changing nature of agent roles may require new hiring profiles and compensation structures to attract and retain talent with the necessary capabilities.
- Ethical workforce transitions: Organizations have varying approaches to handling potential workforce reductions resulting from automation, with implications for corporate reputation and employee relations.
Forward-thinking organizations are proactively addressing these challenges through comprehensive workforce planning, investment in reskilling programs, and thoughtful approaches to managing transitions.
The Path Forward
While these challenges are significant, they are not insurmountable. Organizations that approach AI implementation with clear-eyed recognition of limitations, thoughtful change management, and strategic alignment can realize substantial benefits while avoiding common pitfalls.
The most successful implementations typically share several characteristics:
- Targeted application: Focusing AI on specific use cases where it can deliver clear value rather than attempting comprehensive transformation all at once.
- Human-centered design: Developing solutions that enhance rather than replace human capabilities, with careful attention to both customer and employee experiences.
- Continuous improvement: Establishing feedback mechanisms and regular evaluation processes to identify and address limitations as they emerge.
- Cross-functional governance: Creating oversight structures that bring together technology, operations, compliance, and customer experience perspectives to guide AI strategy.
By acknowledging and proactively addressing these challenges, organizations can develop AI strategies for their call centers that deliver sustainable competitive advantage while managing risks effectively.
Human-AI Collaboration in Call Centers
The Evolution of Agent Roles
The relationship between human agents and AI systems in call centers is undergoing a profound transformation. Rather than a simple narrative of technology replacing people, we are witnessing the emergence of sophisticated collaboration models that leverage the complementary strengths of human and artificial intelligence. This evolution is reshaping agent roles, creating new opportunities for employee development, and fundamentally changing how organizations approach customer service delivery.
Historically, call center agents have been responsible for the entire customer interaction process—from greeting and identification to problem diagnosis, solution delivery, and follow-up. This comprehensive responsibility required broad but often relatively shallow knowledge across multiple domains, with agents frequently consulting scripts, knowledge bases, and supervisors to address complex issues. Performance was typically measured through metrics like average handling time, first call resolution, and customer satisfaction scores.
In today’s AI-enhanced environment, this traditional role is evolving into more specialized and higher-value functions. As AI systems increasingly handle routine transactions and information gathering, human agents are becoming specialized problem solvers, emotional intelligence experts, and relationship builders. This shift represents not just a change in day-to-day activities but a fundamental elevation of the agent role within the customer experience ecosystem.
Models of Human-AI Collaboration
Several distinct models of human-AI collaboration have emerged in call centers, each with different implications for customer experience, operational efficiency, and workforce development:
The Triage Model
In this approach, AI systems serve as the first point of contact for all customer interactions, handling routine inquiries independently and routing more complex issues to appropriate human agents. This model is characterized by:
- AI-powered initial assessment: Virtual assistants or chatbots conduct preliminary conversations to understand customer needs and collect relevant information.
- Intelligent routing: Based on issue complexity, customer profile, and agent expertise, AI systems direct interactions to the most appropriate human resource.
- Contextual handover: When transferring to a human agent, the AI provides comprehensive context about the customer’s history and current needs, eliminating the frustration of repetition.
Financial institutions in the United States and Canada have been particularly successful with this model. A major North American bank reported that implementing AI triage reduced average handling time by 40% while improving customer satisfaction scores, as customers with simple needs received immediate assistance while those with complex issues were quickly connected to appropriately skilled agents.
The Augmented Agent Model
This increasingly popular approach keeps human agents at the center of customer interactions but enhances their capabilities through real-time AI assistance. Key elements include:
- Real-time guidance: AI systems analyze customer conversations as they occur, providing agents with suggested responses, relevant information, and next-best-action recommendations.
- Automated documentation: AI handles note-taking and system updates, allowing agents to focus fully on customer communication rather than administrative tasks.
- Performance feedback: Agents receive immediate feedback on their communication style, empathy, and effectiveness, enabling continuous improvement.
This model has gained significant traction in complex service environments like healthcare and technical support. A major telecommunications provider in Mexico implemented augmented agent technology and saw a 23% improvement in first-call resolution rates and a 15% reduction in average handling time, as agents could access relevant information without placing customers on hold or transferring calls.
The Hybrid Interaction Model
This sophisticated approach dynamically allocates responsibilities between AI and human agents within a single customer interaction, creating a seamless experience that leverages the strengths of both:
- Fluid transitions: Customers may move between AI and human assistance multiple times during a single interaction, based on the specific tasks being performed.
- Simultaneous collaboration: In some implementations, AI and human agents work simultaneously, with the AI handling routine aspects (e.g., data entry, knowledge retrieval) while the human focuses on relationship building and complex decision-making.
- Continuous learning: The AI system learns from observing human agent responses to complex situations, gradually expanding its capabilities.
This model is particularly effective in industries with complex products and high-value customer relationships. A wealth management firm implemented a hybrid interaction system where AI handled account verification, transaction processing, and basic information requests, while human advisors focused on financial guidance and relationship development. The firm reported a 35% increase in advisor productivity and significantly improved client satisfaction.
The Rise of the “Super-Agent”
The integration of AI into call centers is giving rise to what industry analysts have termed the “super-agent”—customer service professionals who leverage AI capabilities to deliver exceptional experiences that would be impossible through either human effort or automation alone. These super-agents represent a new paradigm in customer service, characterized by:
Enhanced Capabilities
Super-agents leverage AI to transcend traditional human limitations:
- Comprehensive knowledge access: Instant access to vast knowledge bases, customer histories, and product information enables agents to provide authoritative answers without delays or uncertainty.
- Predictive insight: AI-generated predictions about customer needs and potential solutions allow agents to proactively address issues before customers even articulate them.
- Emotional intelligence augmentation: Sentiment analysis tools help agents recognize and respond appropriately to customer emotions, even in digital channels where visual and auditory cues are absent.
- Multilingual fluency: Real-time translation capabilities enable agents to serve customers in their preferred language, regardless of the agent’s native tongue.
A customer service leader at a global e-commerce company described the transformation: “Our agents are now able to focus on what humans do best—building rapport, showing empathy, and creative problem-solving—while AI handles the cognitive heavy lifting of retrieving information and processing transactions. The result is a level of service that neither humans nor AI could deliver independently.”
Evolving Skill Requirements
The emergence of super-agents is driving significant changes in the skills and capabilities required for call center roles:
- Technical fluency: Agents need sufficient technical understanding to collaborate effectively with AI systems, interpreting recommendations and recognizing when to override automated suggestions.
- Critical thinking: As routine decision-making becomes automated, human agents must excel at evaluating complex situations, identifying unusual patterns, and developing creative solutions.
- Emotional intelligence: The ability to recognize, understand, and respond appropriately to customer emotions becomes increasingly central to the human agent’s role.
- Adaptability: Agents must be comfortable with continuous change as AI capabilities evolve and their own roles transform accordingly.
These evolving requirements are reshaping hiring profiles for call center positions. Organizations are increasingly seeking candidates with higher education levels, stronger critical thinking skills, and greater emotional intelligence, offering higher compensation to attract and retain this talent.
Career Progression and Specialization
The super-agent model is creating new career paths within customer service organizations:
- AI trainers: Experienced agents who specialize in training and refining AI systems based on their customer service expertise.
- Escalation specialists: Highly skilled agents who handle only the most complex or sensitive customer situations that AI cannot address.
- Experience designers: Professionals who work at the intersection of customer experience, technology, and operations to design optimal human-AI collaboration workflows.
- Analytics specialists: Roles focused on analyzing interaction data to identify improvement opportunities in both human and AI performance.
These specialized roles often offer higher compensation and greater career development opportunities than traditional agent positions, helping organizations attract and retain talented individuals who might previously have viewed call center work as a temporary stepping stone rather than a career path.
Employee Reskilling and Development
The transformation of agent roles necessitates comprehensive approaches to employee development and transition management:
Reskilling Programs
Leading organizations are implementing structured programs to help existing employees develop the skills needed for AI-enhanced roles:
- Technical literacy: Training on AI capabilities, limitations, and effective collaboration techniques.
- Advanced communication: Development of sophisticated communication skills that differentiate human interactions from automated ones.
- Complex problem-solving: Structured approaches to addressing unusual or multifaceted customer issues that fall outside standard procedures.
- Emotional intelligence: Techniques for recognizing and responding to customer emotions, particularly in challenging situations.
A major insurance company in the Philippines implemented a six-month reskilling program for its call center workforce as part of its AI transformation initiative. The program combined online learning, classroom training, and on-the-job coaching, with agents gradually transitioning to AI-augmented roles. The company retained 87% of its existing workforce through this transition, significantly reducing recruitment and onboarding costs while preserving valuable institutional knowledge.
Continuous Learning Models
The dynamic nature of AI technology requires new approaches to ongoing employee development:
- Micro-learning: Short, focused learning modules delivered within the flow of work to help agents adapt to evolving AI capabilities.
- Peer learning communities: Structured opportunities for agents to share experiences and best practices in working with AI systems.
- Simulation-based training: Safe environments where agents can practice handling complex scenarios with AI assistance before facing similar situations with actual customers.
- Performance analytics: Data-driven insights that help agents understand their strengths and development opportunities in the context of human-AI collaboration.
These continuous learning approaches recognize that the skills required for effective human-AI collaboration will continue to evolve as the technology advances, requiring ongoing adaptation rather than one-time training initiatives.
Measuring Success in Human-AI Collaboration
As call centers transition to collaborative human-AI models, traditional performance metrics must evolve to capture the value created through this new approach:
Balanced Scorecard Approaches
Leading organizations are implementing multidimensional measurement frameworks that consider:
- Efficiency metrics: Traditional measures like average handling time and cost per interaction remain relevant but are balanced with other considerations.
- Quality indicators: Comprehensive evaluations of interaction quality, including accuracy, completeness, and appropriateness of responses.
- Customer impact: Measures of customer satisfaction, effort, loyalty, and business outcomes resulting from service interactions.
- Employee experience: Assessment of agent satisfaction, engagement, and development in AI-enhanced roles.
Value Attribution Models
Sophisticated analytics approaches help organizations understand the relative contribution of human and AI elements to overall performance:
- Interaction pattern analysis: Examination of how different combinations of human and AI involvement affect customer outcomes.
- Counterfactual modeling: Estimation of what would have happened in alternative scenarios (e.g., human-only or AI-only handling).
- Longitudinal impact assessment: Evaluation of how human-AI collaboration affects long-term customer relationships and lifetime value.
These measurement approaches help organizations continuously refine their collaboration models, identifying opportunities to optimize the division of responsibilities between human agents and AI systems.
The Future of Human-AI Collaboration
As AI capabilities continue to advance, we can expect further evolution in human-AI collaboration models. Several emerging trends are likely to shape this future:
- Proactive service models: Combined human-AI systems that anticipate customer needs and initiate outreach before problems occur, fundamentally changing the nature of customer service from reactive to proactive.
- Emotion-adaptive interfaces: AI systems that recognize and respond to customer emotions, adjusting their approach and potentially bringing in human agents when emotional support is needed.
- Agent-directed automation: Interfaces that give human agents greater control over when and how AI assistance is deployed, allowing for more nuanced collaboration.
- Collective intelligence systems: Platforms that aggregate insights from multiple human agents and AI systems to continuously improve service delivery across the organization.
These developments suggest that the future of call centers lies not in a binary choice between human service and automation, but in increasingly sophisticated collaboration models that leverage the unique strengths of both. Organizations that excel in designing and implementing these collaborative approaches will create significant competitive advantage through superior customer experience and operational efficiency.
Emerging Trends and Technologies in Call Centers
Generative AI: The Next Frontier
Generative AI represents one of the most transformative technologies emerging in the call center space. Unlike traditional rule-based systems that select from predefined responses, generative AI can create original, contextually appropriate content in real-time. This capability is revolutionizing how call centers handle customer interactions across channels.
GPT-Powered Virtual Agents
Large language models like GPT (Generative Pre-trained Transformer) are enabling a new generation of virtual agents with unprecedented capabilities:
- Conversational fluency: These systems can maintain natural, flowing conversations that closely mimic human communication patterns, moving beyond the stilted interactions characteristic of earlier chatbots.
- Contextual understanding: Advanced generative AI can maintain context throughout complex conversations, remembering previous statements and building coherent responses that acknowledge this history.
- Creative problem-solving: Rather than being limited to predefined solutions, generative AI can synthesize novel approaches to customer issues by drawing on broad knowledge bases and pattern recognition.
- Personalization at scale: These systems can adapt their communication style, level of detail, and recommendations based on individual customer profiles and interaction histories.
By 2025, industry analysts predict that generative AI could handle up to 70% of customer interactions without human intervention in certain industries. Financial institutions are at the forefront of this trend, with several major banks deploying GPT-powered virtual agents that can discuss complex products, troubleshoot account issues, and even provide personalized financial advice within regulatory parameters.
However, this technology also presents significant challenges. Concerns about “hallucinations” (AI generating plausible but incorrect information), data privacy, and appropriate oversight remain important considerations for organizations implementing generative AI in customer-facing roles. Leading organizations are addressing these concerns through robust verification mechanisms, human oversight, and clear escalation paths for complex or sensitive issues.
Multimodal AI: Beyond Text and Voice
While early AI systems in call centers focused primarily on text or voice interactions, multimodal AI represents a significant advancement by integrating multiple communication channels and data types:
- Visual processing: AI systems that can interpret images, documents, and video, allowing customers to show rather than describe problems.
- Voice, text, and visual integration: Seamless transitions between communication modes within a single interaction, accommodating customer preferences and situational needs.
- Sentiment and emotion recognition: Advanced systems that analyze facial expressions, voice tonality, and text sentiment to gauge customer emotions and adjust responses accordingly.
- Gesture and behavior interpretation: Emerging capabilities to understand non-verbal cues in video interactions, further enhancing the system’s ability to respond appropriately.
A leading telecommunications provider has implemented a multimodal AI system that allows customers to troubleshoot device issues through a combination of text chat, voice conversation, and camera sharing. The system can guide customers through diagnostic procedures, visually identify hardware problems, and seamlessly transfer to human agents when necessary, all while maintaining conversation context across modalities.
The integration of these capabilities creates more natural and efficient customer experiences by allowing interactions to occur through the most appropriate channels for specific tasks. For example, a customer might begin with a text chat about a billing question, switch to voice for a more complex explanation, and then use image sharing to clarify a specific document detail—all within a single, continuous interaction.
AI-Driven Quality Assurance and Performance Management
Traditional quality assurance in call centers typically relied on manual review of a small sample of interactions, creating significant limitations in coverage, consistency, and timeliness. AI is transforming this approach through comprehensive, real-time analysis of customer interactions across channels.
100% Interaction Coverage
AI-powered quality management systems can analyze every customer interaction—whether voice, chat, email, or social media—providing unprecedented visibility into service quality and compliance:
- Automated scoring: AI systems evaluate interactions against predefined quality criteria, generating consistent scores without human bias.
- Pattern identification: Advanced analytics identify trends and anomalies across thousands of interactions, highlighting systemic issues that might be missed in sample-based approaches.
- Real-time monitoring: Rather than retrospective review, AI can flag potential quality or compliance issues during live interactions, enabling immediate intervention when necessary.
- Cross-channel consistency: Unified quality standards can be applied across all communication channels, ensuring consistent customer experiences regardless of how they choose to engage.
Organizations implementing these systems report significant improvements in both operational metrics and customer satisfaction. A major healthcare insurer achieved a 35% reduction in compliance-related issues and a 28% improvement in first-call resolution after implementing AI-powered quality management across its contact centers.
Personalized Coaching and Development
Beyond monitoring, AI is revolutionizing how agents receive feedback and develop their skills:
- Individualized insights: AI analysis identifies each agent’s specific strengths and development areas based on their actual interactions rather than generic training programs.
- Micro-learning recommendations: Systems automatically suggest targeted learning resources addressing specific skill gaps identified in recent interactions.
- Simulation-based practice: AI-powered role-play environments allow agents to practice handling challenging scenarios with immediate feedback.
- Performance prediction: Advanced analytics can forecast agent performance trends, enabling proactive coaching before issues impact customer experience.
These capabilities are transforming the supervisor role from reactive monitoring to proactive coaching and development. A financial services organization in India reported a 40% reduction in agent attrition after implementing an AI-powered coaching system that provided personalized development paths and recognition of improvement.
Digital Twins and Simulations in Training
The concept of digital twins—virtual replicas of physical systems—is being applied to call center operations to enhance training, scenario planning, and process optimization.
Agent Training Environments
Digital twin technology creates realistic simulation environments for agent training:
- Scenario-based learning: Agents can practice handling complex customer situations in a risk-free environment before encountering them with actual customers.
- Personalized difficulty scaling: Training scenarios automatically adjust in complexity based on the agent’s skill level and development needs.
- Real-world variation: Simulations incorporate the full range of customer personalities, communication styles, and issue complexities that agents will encounter in their roles.
- Immediate feedback: AI provides real-time guidance during simulations, helping agents develop effective approaches to challenging situations.
A major retail organization implemented digital twin training for seasonal customer service staff and reported a 45% reduction in time-to-proficiency compared to traditional training methods. The system allowed new agents to experience a month’s worth of customer scenarios in just a few days of intensive simulation-based learning.
Process Optimization
Beyond training, digital twins enable organizations to test process changes and technology implementations in a virtual environment before deploying them in production:
- Workflow simulation: Organizations can model how changes to routing rules, knowledge base structures, or automation will affect key performance indicators.
- “What-if” scenario testing: Call center leaders can evaluate how their operation would respond to volume spikes, system outages, or other challenging conditions.
- Technology evaluation: New AI capabilities can be tested in a realistic simulation environment to identify integration issues or unexpected consequences before customer-facing deployment.
These applications help organizations reduce the risk associated with operational changes while accelerating innovation cycles. A telecommunications provider in Mexico used digital twin simulation to optimize its IVR design, testing multiple configurations with simulated customer interactions before identifying an approach that reduced abandonment rates by 23%.
Voice Cloning and Synthetic Speech
Advances in voice synthesis technology are creating new possibilities for personalized and efficient customer communications.
Personalized Voice Responses
Voice cloning technology allows organizations to create consistent, branded voice experiences:
- Brand voice consistency: Organizations can develop distinctive synthetic voices that align with their brand identity and maintain consistency across all voice interactions.
- Emotional calibration: Synthetic voices can be designed with appropriate emotional qualities for different types of customer interactions, from energetic and upbeat for sales to calm and reassuring for support.
- Accent adaptation: Voice systems can adjust accent and speech patterns based on regional preferences while maintaining clear pronunciation and comprehensibility.
Several major airlines have implemented personalized voice response systems that maintain consistent brand voice across all automated communications while adjusting tone and pacing based on the nature of the message—whether confirming a routine reservation or addressing a flight cancellation.
Agent Voice Augmentation
Beyond fully automated systems, voice technology is being used to enhance human agent capabilities:
- Voice rest and preservation: Agents with voice strain can leverage synthetic speech while continuing to manage the substance of customer conversations.
- Accent neutralization: In global call centers, voice technology can help standardize pronunciation while preserving the agent’s control over conversation content.
- Voice transformation: In some applications, voice technology allows male agents to present as female voices or vice versa based on operational needs or customer preferences.
These applications remain somewhat controversial, raising questions about transparency and authenticity in customer interactions. Organizations implementing such technologies must carefully consider ethical implications and appropriate disclosure to customers.
Predictive Customer Intelligence
The integration of advanced analytics, machine learning, and vast data resources is enabling increasingly sophisticated prediction of customer needs and behaviors.
Anticipatory Service Models
Rather than waiting for customers to report problems, predictive systems enable proactive outreach:
- Issue prediction: AI systems analyze patterns in device performance, account activity, or service usage to identify potential problems before they affect customers.
- Churn risk identification: Behavioral indicators and sentiment analysis help identify customers at risk of defection in time for effective intervention.
- Life event recognition: Changes in transaction patterns or communication content may signal important life events (moving, marriage, etc.) that create new service needs.
- Next-best-action determination: Systems predict which offers, solutions, or communications will be most relevant to each customer’s current situation.
A major cable and internet provider implemented predictive service technology that identifies potential network connectivity issues at the household level. The system automatically initiates proactive communication with affected customers, offering troubleshooting guidance or service appointments before many customers even notice a problem. This approach has reduced inbound support calls by 18% while significantly improving customer satisfaction scores.
Journey Orchestration
Advanced predictive systems are moving beyond point-in-time recommendations to orchestrate entire customer journeys:
- Cross-channel consistency: AI ensures that customer information and context follow them across channels and interactions, creating coherent experiences regardless of how they engage.
- Optimal timing determination: Systems identify the best moments for proactive outreach based on customer preferences and receptivity patterns.
- Channel optimization: AI determines which communication channels will be most effective for specific customers and message types.
- Personalized journey mapping: Rather than forcing customers through standardized journeys, AI creates dynamically optimized paths based on individual needs and behaviors.
Financial institutions have been early adopters of journey orchestration technology. A major retail bank implemented an AI-driven orchestration platform that coordinates communications across its mobile app, website, call center, and branch network. The system ensures that customers receive consistent, contextually appropriate service regardless of how they choose to engage, resulting in a 31% improvement in product adoption rates and a 24% reduction in service costs.
The Convergence of Technologies
While each of these emerging technologies offers significant value independently, their true transformative potential lies in their convergence within integrated customer experience platforms. Organizations at the forefront of call center innovation are implementing ecosystems where generative AI, multimodal capabilities, predictive analytics, and simulation technologies work together seamlessly.
In these advanced environments:
- Generative AI creates natural, contextually appropriate responses across channels
- Multimodal systems ensure seamless transitions between communication methods
- Predictive analytics anticipate customer needs and optimal next steps
- Digital twins continuously optimize processes and agent capabilities
- Voice technology creates consistent, emotionally appropriate audio experiences
This convergence is creating unprecedented capabilities for personalization, efficiency, and customer satisfaction. However, it also raises important questions about data privacy, algorithmic transparency, and the appropriate balance between automation and human connection—topics that will be explored in the following section on ethical considerations.
As these technologies continue to mature and become more accessible, we can expect their adoption to accelerate across the call center industry. Organizations that thoughtfully implement these capabilities while addressing associated challenges will create significant competitive advantage through superior customer experiences, operational efficiency, and employee enablement.
The Ethical and Social Dimension of AI in Call Centers
Job Displacement and Workforce Evolution
The integration of AI into call centers raises profound questions about the future of work in this sector. With millions of people employed in call centers globally, the potential for significant job displacement is a legitimate concern that organizations and society must address.
The Scale of Potential Displacement
Research from Oxford University estimates that approximately 47% of U.S. employment is at high risk of automation in the coming decades, with customer service roles particularly vulnerable. In call centers specifically, routine tasks like information provision, basic troubleshooting, and transaction processing—which can constitute 60-70% of current agent activities—are increasingly being automated through AI systems.
This trend is particularly significant in countries where call centers represent a substantial portion of the formal economy. In the Philippines, where the BPO industry employs approximately 1.3 million people and accounts for around 7% of GDP, the automation of call center functions could have far-reaching economic and social implications. Similarly, in India, where the IT-BPM sector employs over 4.5 million people, the evolution of call center roles presents both opportunities and challenges.
Workforce Transition Approaches
Organizations are adopting various approaches to managing this transition:
- Reskilling and upskilling: Proactive companies are investing in comprehensive programs to help existing employees develop the skills needed for evolving roles in AI-enhanced environments. These programs focus on developing capabilities in areas like complex problem-solving, emotional intelligence, and technology collaboration that complement rather than compete with AI.
- Natural attrition management: Some organizations are leveraging the traditionally high turnover rates in call centers (often 30-45% annually) to gradually reduce headcount through attrition rather than layoffs as they implement automation.
- Role redesign: Forward-thinking companies are creating new positions that leverage the complementary strengths of humans and AI, such as AI trainers, escalation specialists, and experience designers.
- Transition support: When displacement is unavoidable, responsible organizations provide comprehensive support including advance notice, severance packages, career counseling, and placement assistance.
The approach organizations take to workforce transition not only affects individual employees but also shapes their reputation as employers and their relationship with the communities in which they operate. Companies recognized for ethical approaches to AI-driven workforce transformation, such as AT&T’s Future Ready initiative which has invested over $250 million in employee reskilling, often see benefits in employee loyalty, public perception, and customer relationships.
Broader Economic Considerations
Beyond individual companies, the automation of call center functions raises important questions about economic distribution and opportunity:
- Geographic impact: Regions that have built significant portions of their economy around call centers and BPO operations may face particular challenges in managing this transition.
- Educational alignment: Educational systems must evolve to prepare workers for the changing nature of customer service roles, emphasizing skills that complement rather than compete with AI.
- Economic inequality: The benefits of AI automation—increased productivity, reduced costs, improved efficiency—flow primarily to technology owners and shareholders, while displacement costs are borne by workers, potentially exacerbating economic inequality.
- New opportunity creation: While certain roles may disappear, AI is also creating new job categories and opportunities that did not previously exist, though these often require different skills and may not be accessible to all displaced workers.
Addressing these broader economic considerations requires collaboration between businesses, educational institutions, governments, and civil society to ensure that the benefits of technological advancement are broadly shared.
Bias in AI and Fairness in Customer Interactions
AI systems can inherit and amplify biases present in their training data, potentially leading to unfair treatment of certain customer groups. This issue is particularly significant in call centers, which serve diverse customer populations and often handle sensitive matters related to financial services, healthcare, and essential utilities.
Sources of Bias in Call Center AI
Bias can enter AI systems used in call centers through various channels:
- Training data imbalances: If historical customer interaction data underrepresents certain demographic groups or contains biased handling patterns, AI systems may perpetuate these imbalances.
- Proxy variables: Even when protected characteristics like race or gender are excluded from models, other variables can serve as proxies, leading to discriminatory outcomes.
- Quality measurement biases: If the metrics used to evaluate “good” customer service reflect cultural or demographic preferences, AI systems optimized for these metrics may serve some groups better than others.
- Voice and language biases: Speech recognition systems often perform better for certain accents, dialects, and speech patterns, potentially creating unequal access to service.
Research from the MIT Media Lab has documented how facial recognition systems demonstrate significantly higher error rates for darker-skinned women compared to lighter-skinned men. Similar disparities have been observed in voice recognition systems, which may have lower accuracy rates for non-native speakers or regional accents.
Consequences of Biased Systems
When AI systems in call centers exhibit bias, the consequences can be significant:
- Unequal access to service: Customers from certain demographic groups may experience longer wait times, more frequent transfers, or lower resolution rates.
- Differential treatment: AI systems might recommend different solutions or offers based on demographic factors rather than legitimate business considerations.
- Reinforcement of stereotypes: Biased systems can perpetuate stereotypical assumptions about different customer groups, affecting how they are perceived and served.
- Legal and regulatory risk: Organizations deploying biased AI systems may face legal liability under anti-discrimination laws and regulations.
These consequences not only harm individual customers but can also damage brand reputation and trust, particularly as awareness of AI ethics issues grows among consumers and regulators.
Approaches to Mitigating Bias
Leading organizations are implementing comprehensive approaches to identify and address bias in their AI systems:
- Diverse and representative training data: Ensuring that the data used to train AI systems adequately represents the full diversity of the customer base.
- Regular bias audits: Systematically testing AI systems for disparate performance or outcomes across different demographic groups.
- Inclusive development teams: Building diverse teams to design, develop, and implement AI systems, bringing varied perspectives to identify potential bias issues.
- Transparent algorithms: Using explainable AI approaches that allow for examination of how decisions are made rather than “black box” systems.
- Human oversight: Maintaining human review of AI recommendations, particularly for high-stakes decisions or edge cases.
Financial services organizations, which face strict regulatory requirements around fair treatment, have been at the forefront of developing robust approaches to bias mitigation. A major U.S. bank implemented a comprehensive bias testing protocol for its virtual assistant, continuously monitoring for disparities in resolution rates and satisfaction scores across demographic groups and making adjustments when issues are identified.
Transparency and Customer Trust
As AI systems take on more significant roles in customer interactions, transparency becomes essential to maintaining trust. Customers have legitimate expectations to understand when they are interacting with AI, how their data is being used, and what recourse they have when issues arise.
Disclosure of AI Use
Organizations must make thoughtful decisions about how to disclose AI involvement in customer interactions:
- Explicit disclosure: Clearly informing customers when they are interacting with an AI system rather than a human agent.
- Capability transparency: Helping customers understand what the AI system can and cannot do to set appropriate expectations.
- Handoff clarity: When interactions transition between AI and human agents, making this transition clear to customers.
Research indicates that customers generally prefer knowing when they are interacting with AI systems. A 2024 survey found that 78% of consumers want explicit disclosure when interacting with AI, and 64% reported feeling uncomfortable when they discovered they had been interacting with AI that had presented itself as human.
Data Usage Transparency
Organizations must also be transparent about how customer data is used in AI systems:
- Purpose specification: Clearly explaining why customer information is being collected and how it will be used.
- Data retention policies: Providing information about how long interaction data will be stored and for what purposes.
- Third-party sharing: Disclosing whether and how customer data may be shared with external organizations.
- AI training usage: Informing customers if their interactions may be used to train or improve AI systems.
These transparency practices not only build trust but also help organizations comply with evolving data protection regulations like GDPR, CCPA, and industry-specific requirements.
Appeal and Redress Mechanisms
When AI systems make decisions that affect customers, organizations should provide clear mechanisms for appeal and redress:
- Human escalation paths: Ensuring customers can reach human agents when they disagree with AI decisions or recommendations.
- Explanation capabilities: Providing understandable explanations for significant AI-driven decisions, particularly in areas like credit, insurance, or service eligibility.
- Correction processes: Establishing clear procedures for correcting errors in AI systems or their underlying data.
These mechanisms acknowledge the limitations of AI systems and demonstrate organizational commitment to fair treatment and customer satisfaction.
Guidelines for Responsible AI Adoption
As organizations navigate the ethical complexities of AI implementation in call centers, several frameworks and guidelines have emerged to promote responsible practices.
Regulatory Developments
Government and regulatory bodies are increasingly establishing requirements for AI use:
- The TCPA One-to-One Consent Rule: Effective January 2025, this rule requires explicit, individualized consent for each seller using automated calling systems.
- FCC’s new rules on robocalls and robotexts: These regulations make it easier for consumers to revoke consent and place additional requirements on organizations using automated communication systems.
- Executive Order on AI Safety: Signed in late 2023, this order establishes guidelines for responsible AI development and use, with particular attention to transparency, fairness, and accountability.
- State-specific regulations: States like California, Colorado, and Virginia have enacted comprehensive data privacy laws with implications for AI systems that process customer information.
Organizations must stay abreast of this evolving regulatory landscape to ensure compliance and avoid potential penalties.
Industry Standards and Self-Regulation
Beyond formal regulations, industry groups and standards organizations are developing frameworks for ethical AI use:
- IEEE Global Initiative on Ethics of Autonomous and Intelligent Systems: This initiative has developed detailed standards for ethically aligned design of AI systems.
- Partnership on AI: This consortium of technology companies, civil society organizations, and academic institutions develops best practices for responsible AI deployment.
- Industry-specific guidelines: Sectors like financial services and healthcare have developed specialized frameworks addressing the unique ethical considerations in their domains.
These voluntary standards often go beyond minimum regulatory requirements to establish best practices that build trust and mitigate risks.
Organizational Ethics Frameworks
Leading organizations are developing comprehensive internal frameworks to guide their AI implementations:
- Ethics review boards: Cross-functional committees that evaluate proposed AI applications against ethical criteria before approval.
- Impact assessments: Structured processes to evaluate the potential consequences of AI systems on various stakeholders, including customers, employees, and communities.
- Monitoring and audit protocols: Ongoing review processes to ensure AI systems continue to operate as intended and align with ethical principles.
- Training and awareness programs: Initiatives to ensure that all employees involved in designing, implementing, or working with AI systems understand relevant ethical considerations.
These organizational frameworks help translate high-level ethical principles into practical decision-making processes that shape day-to-day operations.
Balancing Innovation and Responsibility
The ethical challenges of AI in call centers do not suggest that organizations should avoid innovation. Rather, they highlight the importance of thoughtful, responsible approaches that consider the full range of impacts on customers, employees, and society.
Organizations that successfully navigate these ethical considerations typically share several characteristics:
- Stakeholder engagement: They actively involve diverse stakeholders—including customers, employees, community representatives, and ethics experts—in shaping their AI strategy.
- Iterative implementation: They adopt phased approaches that allow for learning and adjustment rather than rushing to implement the most advanced capabilities immediately.
- Outcome monitoring: They establish comprehensive metrics that look beyond operational efficiency to measure the broader impacts of AI systems on customer experience, employee wellbeing, and societal outcomes.
- Continuous learning: They view ethical AI implementation as an ongoing journey rather than a one-time compliance exercise, continuously refining their approach as technology evolves and new insights emerge.
By embracing these practices, organizations can harness the transformative potential of AI in call centers while upholding their responsibilities to customers, employees, and society. The most successful organizations will be those that view ethical considerations not as constraints on innovation but as essential elements of sustainable, trust-based customer relationships in the AI era.
Strategic Recommendations for AI Integration in Call Centers
Building a Roadmap for AI Transformation
Successful AI integration in call centers requires a structured approach that balances technological possibilities with organizational realities. The following framework provides a roadmap for organizations at various stages of their AI journey.
Assessment and Vision Development
Before implementing specific AI solutions, organizations should conduct a comprehensive assessment of their current state and develop a clear vision for their future:
- Current state analysis: Evaluate existing call center operations, including technology infrastructure, workforce capabilities, customer experience metrics, and operational efficiency.
- Pain point identification: Identify specific challenges that AI could address, such as high call volumes, lengthy handling times, inconsistent service quality, or agent turnover.
- Customer journey mapping: Document current customer journeys across channels, highlighting moments of friction or dissatisfaction that could benefit from AI enhancement.
- Vision articulation: Develop a clear, compelling vision for how AI will transform the customer and employee experience, aligned with broader organizational strategy and brand positioning.
This foundational work ensures that subsequent AI investments address genuine business needs rather than implementing technology for its own sake. A major insurance company began its AI transformation by mapping over 200 distinct customer journeys, identifying specific friction points where AI could create the most significant impact, and establishing clear success metrics for each intervention.
Prioritization and Roadmap Development
With a clear understanding of current state and future vision, organizations can develop a prioritized implementation roadmap:
- Quick win identification: Identify high-impact, low-complexity opportunities that can deliver rapid results and build momentum for the transformation journey.
- Capability building sequencing: Plan the logical progression of AI capabilities, recognizing dependencies and building blocks that enable more advanced applications.
- Risk-based prioritization: Consider implementation risks, including technical complexity, change management challenges, and potential customer impact, when sequencing initiatives.
- Resource alignment: Ensure that the implementation roadmap aligns with available financial, technical, and human resources, avoiding overcommitment that could lead to failed initiatives.
Effective roadmaps typically balance short-term operational improvements with longer-term strategic capabilities. A telecommunications provider in Canada developed a three-horizon roadmap: the first focused on automating simple customer inquiries through virtual assistants; the second on implementing agent assistance tools; and the third on predictive service models that could anticipate and prevent customer issues.
Technology Selection and Integration
Choosing the right AI technologies and partners is critical to successful implementation:
- Build vs. buy decisions: Determine which capabilities should be developed internally versus procured from vendors, based on strategic importance, internal capabilities, and resource constraints.
- Vendor evaluation criteria: Develop comprehensive criteria for evaluating potential technology partners, including technical capabilities, industry expertise, implementation support, and cultural alignment.
- Integration architecture: Design a flexible architecture that allows for integration of multiple AI capabilities while minimizing technical debt and avoiding vendor lock-in.
- Data strategy: Develop a comprehensive approach to data collection, management, and governance that supports current and future AI applications while ensuring compliance with privacy regulations.
Organizations should resist the temptation to select technologies based solely on advanced features or market hype. A healthcare payer that initially selected the most sophisticated virtual assistant platform available later switched to a simpler solution that better integrated with their existing systems and provided more straightforward implementation support.
Change Management and Workforce Transformation
The human dimensions of AI implementation are often more challenging than the technical aspects:
- Stakeholder engagement: Identify and engage key stakeholders, including frontline agents, supervisors, customers, and cross-functional partners, throughout the transformation journey.
- Communication strategy: Develop clear, consistent messaging about the purpose and impact of AI implementation, addressing concerns and managing expectations.
- Training and development: Create comprehensive programs to help employees develop the skills needed to work effectively with AI systems and transition to evolving roles.
- Performance management alignment: Update performance metrics, incentives, and career paths to reflect the changing nature of work in an AI-enhanced environment.
Organizations that excel in change management typically involve employees in the design process rather than imposing solutions from above. A BPO provider in the Philippines created an “AI ambassador” program, selecting high-performing agents to participate in technology selection, process design, and training development, creating internal champions who helped drive adoption.
Governance and Continuous Improvement
Sustainable AI transformation requires robust governance and ongoing optimization:
- Cross-functional oversight: Establish governance structures that bring together perspectives from operations, technology, compliance, customer experience, and human resources.
- Ethical guidelines: Develop clear principles and review processes to ensure AI implementations align with organizational values and ethical standards.
- Measurement framework: Implement comprehensive metrics that capture both operational impacts and broader outcomes for customers, employees, and the business.
- Feedback mechanisms: Create structured processes to gather insights from customers and employees about AI performance and opportunities for improvement.
These governance mechanisms help organizations maintain strategic alignment as they scale AI implementations across the enterprise. A financial services organization established a quarterly “AI ethics review” where cross-functional leaders evaluated the performance of AI systems against established ethical principles and identified potential issues requiring attention.
Selecting the Right AI Partners and Technologies
The rapidly evolving AI landscape presents organizations with a complex array of potential technology partners and solutions. Making informed selection decisions requires a structured approach.
Capability Assessment Framework
Organizations should evaluate potential AI solutions against a comprehensive capability framework:
- Functional capabilities: The specific features and functions the solution provides, such as natural language understanding, sentiment analysis, or predictive analytics.
- Technical architecture: The underlying technology approach, including cloud versus on-premises deployment, integration capabilities, and scalability.
- Implementation requirements: The resources, timeline, and organizational changes needed to successfully deploy the solution.
- Ongoing management: The effort and expertise required to maintain, optimize, and evolve the solution over time.
This structured assessment helps organizations look beyond marketing claims to understand the practical implications of different technology choices. A retail organization developed a detailed capability scorecard for evaluating virtual assistant platforms, weighting different criteria based on their specific requirements and using this framework to conduct a rigorous, objective evaluation.
Vendor Ecosystem Considerations
Beyond individual technology capabilities, organizations should consider the broader ecosystem in which potential partners operate:
- Integration partnerships: The vendor’s relationships with other technology providers, particularly those in the organization’s existing ecosystem.
- Industry expertise: The vendor’s experience and knowledge in the organization’s specific industry, including relevant use cases and compliance requirements.
- Innovation roadmap: The vendor’s vision for future development and their track record of delivering on promised innovations.
- Financial stability: The vendor’s business model, funding status, and long-term viability, particularly important for startups or newer entrants.
These ecosystem factors can significantly impact the success and sustainability of AI implementations. A healthcare organization initially selected an AI provider based on advanced technical capabilities but later encountered challenges because the vendor lacked healthcare-specific expertise and pre-built integrations with clinical systems.
Implementation and Support Models
The approach to implementation and ongoing support is as important as the technology itself:
- Implementation methodology: The vendor’s approach to project management, configuration, integration, and change management.
- Training and enablement: The resources and support provided to help the organization’s team develop necessary skills and knowledge.
- Ongoing optimization: The vendor’s approach to continuous improvement, including how they incorporate feedback and measure success.
- Support model: The structure, availability, and expertise of the vendor’s support organization, particularly for mission-critical applications.
Organizations should thoroughly evaluate these aspects through detailed discussions, reference checks, and pilot implementations. A telecommunications provider in Mexico implemented a structured “proof of concept” approach with three potential AI partners, evaluating not just the technology but also the quality of implementation support and responsiveness to feedback.
Build vs. Buy Considerations
For some organizations, developing proprietary AI capabilities may be preferable to purchasing commercial solutions:
- Strategic differentiation: When AI capabilities directly enable unique customer experiences or competitive advantage, internal development may be warranted.
- Specialized requirements: Organizations with highly specific needs that aren’t well-served by commercial solutions may need custom development.
- Data sensitivity: When working with particularly sensitive data or in highly regulated environments, internal development may provide greater control.
- Existing capabilities: Organizations with strong data science and engineering teams may leverage these resources for internal development.
However, internal development requires significant investment in specialized talent, infrastructure, and ongoing maintenance. A major financial institution initially attempted to build a proprietary virtual assistant but later shifted to a commercial platform after recognizing the substantial resources required to keep pace with rapidly evolving language models and conversational capabilities.
Governance Frameworks and KPIs to Track
Effective governance ensures that AI implementations deliver intended benefits while managing risks appropriately. A comprehensive governance framework should address multiple dimensions.
Performance Metrics
Organizations should track a balanced set of metrics to evaluate AI performance:
- Operational efficiency: Measures like containment rate (percentage of interactions handled without human intervention), average handling time, and cost per interaction.
- Customer experience: Metrics including customer satisfaction, effort scores, net promoter score, and sentiment analysis results.
- Employee impact: Indicators such as agent satisfaction, turnover rates, and productivity measures.
- Business outcomes: Revenue generation, cost savings, customer retention, and other financial and strategic impacts.
The specific metrics will vary based on organizational priorities and the nature of AI implementations. A retail bank developed a balanced scorecard for its virtual assistant implementation, weighting different metrics based on strategic priorities and tracking trends over time rather than focusing solely on absolute values.
Risk Management Framework
AI implementations introduce various risks that require structured monitoring and mitigation:
- Compliance risks: Potential violations of regulations related to data privacy, consumer protection, or industry-specific requirements.
- Operational risks: Possibilities of system failures, performance degradation, or security breaches.
- Reputational risks: Potential damage to brand perception due to AI errors, biased outcomes, or customer frustration.
- Ethical risks: Concerns related to fairness, transparency, and responsible use of AI technologies.
Organizations should establish clear risk thresholds, monitoring processes, and escalation procedures for each risk category. A healthcare organization implemented a “risk radar” that continuously monitored its AI systems for potential issues, with automated alerts when key risk indicators approached predefined thresholds.
Continuous Improvement Processes
Governance should include structured approaches to ongoing optimization:
- Performance review cadence: Regular meetings to evaluate AI performance against established metrics and identify improvement opportunities.
- Customer feedback integration: Systematic processes to collect and analyze customer input about AI interactions.
- Agent feedback channels: Mechanisms for frontline employees to report issues and suggest enhancements.
- A/B testing framework: Structured approaches to testing potential improvements before full implementation.
These processes ensure that AI systems evolve based on real-world performance rather than remaining static after initial deployment. A telecommunications provider established a monthly “AI optimization forum” where cross-functional teams reviewed performance data, customer feedback, and agent input to prioritize enhancements to their virtual assistant.
Ethical Oversight
Given the significant ethical implications of AI in customer service, specialized governance mechanisms are essential:
- Ethics committee: A dedicated group with diverse perspectives to evaluate AI implementations against ethical principles.
- Bias monitoring: Regular assessment of AI outcomes across different customer segments to identify potential disparities.
- Transparency reviews: Periodic evaluation of how AI use is disclosed to customers and whether these disclosures are effective.
- Human oversight mechanisms: Clear processes for human review of AI decisions, particularly in high-stakes situations.
These ethical governance mechanisms help organizations maintain alignment with their values and build sustainable trust with customers and employees. A financial services organization established an “AI ethics council” with representatives from legal, compliance, customer experience, diversity and inclusion, and frontline operations, meeting quarterly to review AI performance and address emerging ethical considerations.
Preparing for the Future
As AI technologies continue to evolve rapidly, organizations must balance current implementation with preparation for future capabilities.
Technology Horizon Scanning
Organizations should establish systematic processes to monitor emerging AI technologies and assess their potential impact:
- Research partnerships: Relationships with academic institutions, research organizations, or technology incubators to gain early insight into emerging capabilities.
- Vendor innovation programs: Participation in partner advisory boards or early adopter programs to influence development roadmaps and gain early access to new features.
- Competitive intelligence: Structured monitoring of how competitors and adjacent industries are applying new AI capabilities.
- Experimentation budget: Dedicated resources for small-scale pilots of promising new technologies before full-scale implementation.
These horizon scanning activities help organizations anticipate and prepare for technological shifts rather than reacting to them after they’ve become mainstream. A major retailer established an “AI innovation lab” where small teams could experiment with emerging technologies in a controlled environment, evaluating potential applications before making significant investments.
Scenario Planning
Given the uncertainty surrounding AI evolution, organizations should use scenario planning to prepare for multiple possible futures:
- Technology scenarios: Different trajectories for AI capability development, from incremental improvement to transformative breakthroughs.
- Regulatory scenarios: Various potential regulatory environments, from minimal oversight to comprehensive restrictions.
- Customer adoption scenarios: Different patterns of customer acceptance and preference for AI-driven interactions.
- Competitive scenarios: Various ways in which competitors might leverage AI to change industry dynamics.
For each scenario, organizations should identify implications for their strategy, required capabilities, and potential risks. A healthcare organization developed four distinct scenarios for the evolution of AI in patient communication, using these to inform their technology investments, partnership strategies, and workforce planning.
Adaptive Strategy Development
Rather than creating rigid long-term plans, organizations should adopt adaptive approaches that can evolve as the AI landscape changes:
- Modular architecture: Technical designs that allow components to be replaced or enhanced as new capabilities emerge.
- Capability-based planning: Focus on building foundational capabilities that enable flexibility rather than committing to specific technologies.
- Options-based investment: Approach technology investments as creating options for future directions rather than irreversible commitments.
- Regular strategy reviews: Scheduled reassessments of AI strategy based on technology evolution, market changes, and organizational learning.
These adaptive approaches help organizations maintain strategic direction while remaining responsive to a rapidly changing environment. A financial services organization adopted a quarterly “strategy refresh” process for its AI initiatives, systematically evaluating whether emerging technologies or changing customer preferences warranted adjustments to their implementation roadmap.
By implementing these strategic recommendations, organizations can navigate the complex landscape of AI in call centers, creating sustainable competitive advantage through enhanced customer experiences, operational efficiency, and employee enablement. The most successful organizations will be those that view AI not as a one-time technology implementation but as a continuous journey of transformation and adaptation.
The Future Outlook for AI in Call Centers
The 5-10 Year Horizon
As we look toward the future of AI in call centers over the next 5-10 years, several key trends and developments are likely to shape the landscape:
Technological Evolution
The technological capabilities underpinning call center AI will continue to advance at a rapid pace:
- Generative AI maturation: Large language models will become increasingly sophisticated, with enhanced reasoning capabilities, reduced hallucinations, and improved contextual understanding. These advancements will enable virtual agents to handle progressively more complex customer interactions with minimal human oversight.
- Multimodal integration: AI systems will seamlessly blend voice, text, visual, and spatial inputs, creating truly omnichannel experiences that adapt to customer preferences and situational needs. Customers will be able to switch effortlessly between communication modes while maintaining conversation context.
- Emotional intelligence enhancement: AI will develop more nuanced understanding of human emotions through advances in sentiment analysis, facial recognition, and voice tonality interpretation. This will enable more empathetic and personalized customer interactions, even in fully automated exchanges.
- Ambient intelligence: AI systems will become more proactive and contextually aware, anticipating customer needs based on environmental signals, behavioral patterns, and predictive analytics. This shift from reactive to proactive service will fundamentally change how customers experience support.
These technological advancements will continuously expand the range of interactions that can be effectively handled through automation, though the most complex, emotionally charged, or high-stakes situations will likely remain the domain of human agents for the foreseeable future.
Business Model Transformation
The economics and organizational structure of call centers will undergo significant transformation:
- Hybrid workforce optimization: Organizations will develop increasingly sophisticated approaches to blending human and AI capabilities, with dynamic allocation of tasks based on complexity, customer preference, and business value. This will drive both efficiency improvements and enhanced customer experiences.
- Outcome-based pricing: Call center services, whether internal or outsourced, will increasingly be priced based on business outcomes rather than traditional metrics like call volume or handling time. This shift will align incentives around value creation rather than cost minimization.
- Ecosystem integration: Call centers will become more deeply integrated with other business functions, with AI enabling seamless handoffs between service, sales, marketing, and product development. This integration will create more holistic customer experiences and enable more effective closed-loop improvement processes.
- Geographic redistribution: The combination of AI automation and remote work capabilities will reshape the geographic distribution of call center operations, potentially reducing the concentration in traditional outsourcing hubs while creating new opportunities for distributed service delivery models.
These business model changes will require organizations to rethink traditional approaches to call center management, performance measurement, and workforce development.
Customer Expectation Evolution
Customer expectations regarding service interactions will continue to evolve in response to technological capabilities and broader societal trends:
- Personalization as standard: Customers will expect interactions that reflect comprehensive understanding of their history, preferences, and current context, regardless of whether they’re engaging with human agents or AI systems.
- Channel fluidity: The distinction between different service channels will become increasingly blurred from the customer perspective, with expectations of seamless transitions and consistent experiences across touchpoints.
- Time compression: Expectations for immediate response and resolution will intensify, with customers increasingly unwilling to accept delays or friction in service interactions.
- Transparency demands: Customers will expect greater clarity about when they are interacting with AI versus humans, how their data is being used, and what options exist for human escalation when needed.
Organizations that fail to meet these evolving expectations will face significant competitive disadvantages, regardless of the efficiency of their operations.
Regulatory Landscape Development
The regulatory environment surrounding AI in customer service will continue to mature:
- AI-specific legislation: Comprehensive legal frameworks governing AI use in customer interactions will emerge in major markets, addressing issues like transparency, accountability, data usage, and bias mitigation.
- Industry-specific requirements: Regulated industries like financial services, healthcare, and telecommunications will face specialized requirements for AI deployment in customer service contexts, reflecting the unique risks and considerations in these sectors.
- International harmonization efforts: As AI regulation proliferates across jurisdictions, efforts to harmonize approaches will emerge to reduce compliance complexity for global organizations.
- Certification standards: Independent certification frameworks will develop to validate AI systems against ethical, performance, and safety standards, providing both regulatory compliance and market differentiation.
These regulatory developments will create both constraints and opportunities, rewarding organizations that proactively address ethical considerations in their AI implementations.
A Call to Action for Proactive, Responsible Adoption
As AI continues to transform call centers, organizations face a critical choice: they can approach this transformation reactively, implementing technologies in response to competitive pressure or cost imperatives, or they can adopt a proactive, strategic approach that shapes the future of customer experience while addressing broader societal implications.
The Imperative for Strategic Action
Several factors make strategic engagement with AI transformation an imperative rather than an option:
- Competitive dynamics: Organizations that successfully implement AI in their call centers can achieve significant advantages in both cost structure and customer experience quality. Those that delay risk falling behind competitors who can deliver superior experiences at lower costs.
- Workforce implications: The evolution of call center roles will happen regardless of individual organizational choices. Proactive approaches allow for thoughtful transition planning that benefits both employees and employers, while reactive approaches often lead to disruptive changes and talent loss.
- Customer expectations: As customers become increasingly familiar with AI-enhanced service experiences, their expectations will shift accordingly. Organizations that fail to meet these evolving expectations risk customer dissatisfaction and attrition.
- Ethical responsibility: The deployment of AI in customer service raises significant ethical questions that organizations have an obligation to address thoughtfully rather than as an afterthought.
These imperatives suggest that all organizations should be actively developing their approach to AI in call centers, regardless of their current implementation status.
Principles for Responsible Adoption
Organizations seeking to navigate this transformation responsibly should adhere to several core principles:
- Human-centered design: Place customer and employee needs at the center of AI strategy, designing systems that enhance human capabilities and experiences rather than simply replacing human roles.
- Ethical foundation: Establish clear ethical principles to guide AI implementation decisions, addressing issues like transparency, fairness, privacy, and accountability from the outset.
- Inclusive development: Ensure that diverse perspectives—including those of frontline agents, customers from different backgrounds, and traditionally underrepresented groups—inform the design and implementation of AI systems.
- Continuous learning: Approach AI implementation as an ongoing journey of experimentation, evaluation, and adaptation rather than a one-time technology deployment.
- Balanced measurement: Evaluate success through multiple lenses, including operational efficiency, customer experience, employee impact, and societal implications.
Organizations that embrace these principles will be better positioned to realize the benefits of AI while managing associated risks and challenges.
The Path Forward
The transformation of call centers through AI represents one of the most significant applications of artificial intelligence in business today, with implications that extend far beyond operational metrics to fundamental questions about the future of work, customer relationships, and organizational responsibility.
Organizations that approach this transformation thoughtfully—balancing technological possibilities with human needs, short-term efficiency with long-term sustainability, and business objectives with ethical considerations—will not only achieve superior outcomes but will help shape a future where technology enhances rather than diminishes human connection and value creation.
The call center of tomorrow will not be defined solely by the sophistication of its AI systems but by how effectively those systems are integrated into a holistic approach to customer experience that recognizes the enduring importance of human empathy, judgment, and creativity alongside the efficiency, consistency, and scalability that technology provides.
By embracing this balanced vision and taking proactive steps to realize it, organizations can transform their call centers from cost centers into strategic assets that create meaningful value for customers, employees, and the business as a whole. The future of AI in call centers is not predetermined—it will be shaped by the choices organizations make today about how to harness these powerful technologies in service of human needs and aspirations.
PITON-Global connects you with industry-leading outsourcing providers to enhance customer experience, lower costs, and drive business success.
Digital Marketing Champion | Strategic Content Architect | Seasoned Digital PR Executive
Jedemae Lazo is a powerhouse in the digital marketing arena—an elite strategist and masterful communicator known for her ability to blend data-driven insight with narrative excellence. As a seasoned digital PR executive and highly skilled writer, she possesses a rare talent for translating complex, technical concepts into persuasive, thought-provoking content that resonates with C-suite decision-makers and everyday audiences alike.
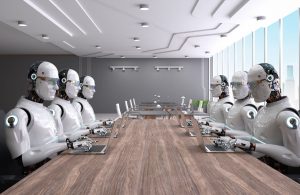
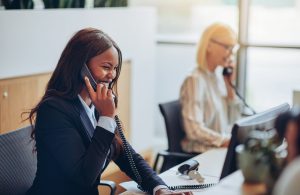
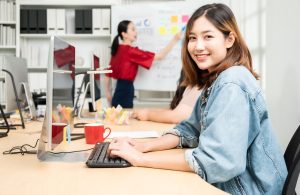